Machine Learning Interatomic Potentials: Development, Datasets, and Benchmarking
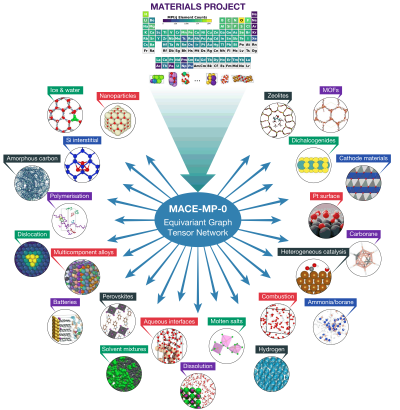
MLIP Development
The rapid growth of MLIPs, trained on millions of density functional theory (DFT) calculations, has demonstrated significant generalization capabilities across diverse material systems. At the Asta group, we have contributed to the development and training of foundation MLIP MACE-MP-0. We are actively collaborating with others to integrate additional physical observables and train MLIPs using higher-level density functionals. Our efforts aim to enhance the predictive power and applicability of MLIPs across challenging materials spaces.
MLIP Benchmarking
Quantitative benchmarks for MLIPs remain limited, hindering robust evaluations of their performance. To address this, we are developing MLIP Arena, a comprehensive benchmarking framework. This benchmark seeks to expand upon previous benchmarking efforts to include off-equilibrium force predictions, deformations to extreme pressures, stability assessments, speed tests, and so on. This ongoing effort will provide a fair and transparent platform for standardizing MLIP assessments.
MLIP Dataset Generation
Until recently, universal MLIPs—potentials spanning most or all elements in the periodic table—have traditionally relied on datasets not explicitly designed for MLIP training. To bridge this gap, we are creating a novel dataset using advanced sampling techniques optimized for MLIP development. This initiative aims to provide a foundation for generating more reliable and versatile MLIPs in the future. This project is currently in progress.